8 Ethical Considerations for Machine Learning Engineers to Address
In a world increasingly driven by artificial intelligence, the ethical responsibilities of machine learning engineers have never been more crucial. This Q&A article unveils eight key insights, starting with the necessity to address bias and fairness and concluding with the importance of promoting responsible AI use. Each insight underscores a critical aspect of ethical engineering in the realm of machine learning. Read on to discover what experts have to say about these pressing ethical considerations.
- Address Bias and Fairness
- Mitigate Algorithmic Bias
- Tackle Bias in Training Data
- Protect User Privacy
- Ensure Model Transparency
- Prioritize Human Well-Being
- Mitigate Unintended Consequences
- Promote Responsible AI Use
Address Bias and Fairness
Bias and fairness are critical ethical considerations because they directly impact the trustworthiness, equity, and societal consequences of machine-learning systems. Machine-learning models are often deployed in high-stakes areas such as hiring, lending, healthcare, and law enforcement, where biased decisions can reinforce systemic inequalities and harm underrepresented or vulnerable populations.
Public trust in machine-learning systems erodes when unfairness is uncovered. Bias-related incidents can result in damage to reputation, loss of user confidence, and legal consequences for organizations.
Many jurisdictions are introducing regulations to ensure fairness in automated decision-making (e.g., GDPR). Addressing bias proactively helps ML engineers and organizations meet these requirements and avoid penalties.
Engineers building Artificial Intelligence and Machine Learning systems have a duty to ensure that technology serves all segments of society equitably. Ignoring bias undermines the potential for ML to be a force for good and risks amplifying harm.
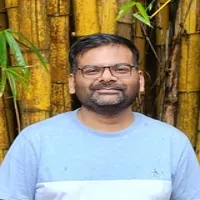
Mitigate Algorithmic Bias
One ethical consideration that is particularly important for machine-learning engineers to address is algorithmic bias. This occurs when AI systems reflect or amplify biases present in the data used to train them, potentially leading to unfair and discriminatory outcomes. As machine learning becomes increasingly integrated into decision-making processes, this issue demands urgent attention.
Algorithmic bias is significant for several reasons:
1. Impact on Individuals and Communities: Biased models can deny access to critical opportunities or services, disproportionately affecting marginalized groups. This not only perpetuates existing inequalities but can also create new forms of discrimination.
2. Lack of Transparency and Accountability: Machine-learning models are often complex and opaque, making it difficult to identify and address sources of bias. This erodes trust in AI systems and the organizations deploying them.
3. Perpetuation of Societal Biases: By reflecting biases in training data, models risk reinforcing harmful stereotypes related to race, gender, and other characteristics, giving the appearance of objectivity to entrenched inequalities.
4. Ethical Responsibility: Engineers have a moral obligation to ensure AI systems are developed and deployed equitably, as their work impacts not just individuals but society at large.
To address algorithmic bias, engineers must take proactive steps, including:
- Auditing Data: Carefully analyze training data for imbalances and diversify data sources to ensure representation.
- Debiasing Models: Apply techniques such as adversarial training, data augmentation, and fairness-aware algorithms.
- Rigorous Testing: Continuously evaluate models to detect and mitigate biases before deployment.
- Collaboration: Engage with domain experts, ethicists, and impacted communities to understand potential harms and context.
- Transparency: Document the development process, employ Explainable AI techniques, and openly communicate system limitations.
Addressing algorithmic bias is not just a technical challenge but a societal imperative. By prioritizing fairness and accountability, machine-learning engineers can ensure AI systems promote equity, uphold public trust, and create a positive impact in the world.
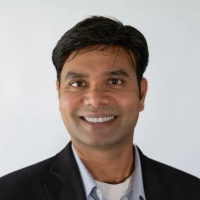
Tackle Bias in Training Data
One ethical consideration that machine-learning engineers must address is bias in training data. Bias in machine learning arises when datasets reflect societal inequalities, historical imbalances, or flawed collection methods. Left unchecked, these biases can be perpetuated or amplified by models, resulting in unfair or harmful outcomes.
Machine-learning models learn patterns from the data they're trained on. If the data underrepresents certain groups or reflects stereotypes, the model will mirror these biases. For example, facial recognition systems have historically struggled with accuracy for people with darker skin tones due to imbalanced training data. Similarly, hiring algorithms have sometimes favored male candidates because of biased historical records, reinforcing gender disparities in workplaces.
This consideration is significant because biased models can harm individuals and exacerbate existing inequalities. In sectors like healthcare, biased models can lead to disparities in treatment recommendations, disproportionately affecting underserved populations. In criminal justice, predictive policing algorithms have reinforced historical biases, unfairly targeting marginalized communities. Such outcomes erode public trust and undermine the ethical use of AI.
Addressing bias is not just a technical necessity but a moral imperative to ensure fairness, inclusivity, and accountability in AI systems. Engineers must actively evaluate their training data, identify potential sources of bias, and use strategies to mitigate it. This includes re-sampling data to improve representation, generating synthetic data, or employing fairness-aware algorithms designed to reduce disparities during model training.
Transparency is also critical. Engineers should communicate a model's limitations and potential risks to stakeholders, empowering them to use AI responsibly. Regularly testing models on diverse datasets helps ensure equitable performance across different groups.
Ultimately, addressing bias is about creating systems that align with ethical principles of justice and equity. As machine-learning systems increasingly influence hiring, lending, healthcare, and policing, prioritizing fairness ensures these tools contribute to a more inclusive and equitable society.

Protect User Privacy
Machine learning engineers have a duty to protect user privacy at each step of the ML lifecycle. They should ensure that data collected is kept secure and is only used for its intended purpose. Maintaining privacy means implementing strong encryption methods and regular security audits.
Trust between users and companies can be strengthened when privacy is given priority. Engineers should actively look for ways to enhance privacy features. Remember, user privacy is key to sustaining long-term trust, so take action now to make it a top priority.
Ensure Model Transparency
Ensuring transparency and explainability of ML models is crucial for machine learning engineers. This means that they should make sure the inner workings of their models are understandable to both experts and non-experts. Transparency helps in building trust and accountability, especially when ML models are used in critical areas like health care or finance.
Explainable AI can help in identifying and correcting biases or errors within the models. Engineers should push for methods and tools that make their models more transparent. Take steps today to make your ML models clear and understandable.
Prioritize Human Well-Being
Prioritizing human well-being and assessing societal impact is essential for ML engineers. When developing models, they must consider how these tools will affect people's daily lives. These engineers should focus on creating solutions that enhance quality of life and do not harm individuals or communities.
Considering the broader impact of ML helps in cultivating ethical practices and public trust. By prioritizing positive societal impact, engineers can contribute to global well-being. Make it a goal to prioritize human well-being in all ML projects.
Mitigate Unintended Consequences
Mitigating unintended consequences and risks is a critical responsibility for machine learning engineers. Unexpected results or misuse of ML can lead to significant ethical and practical problems. Engineers should implement thorough testing and simulation to identify possible negative outcomes before deployment.
By addressing potential risks early, adverse impacts can be minimized. Preparing for unintended consequences enhances the robustness and safety of ML applications. Start implementing risk mitigation strategies in your ML projects right away.
Promote Responsible AI Use
Promoting the responsible use and access to AI is an important task for ML engineers. Ensuring that AI is accessible in an ethical manner means considering who is benefiting from these technologies and who might be disadvantaged. Engineers must work to make sure that AI is not used to deepen inequalities or create unfair advantages.
Responsible use also includes educating stakeholders about ethical AI practices. By promoting fairness and equal access, engineers can help build a just AI ecosystem. Commit to promoting responsible AI use in your future projects.