4 Go-to Resources for Staying Updated On Machine Learning Trends
Staying ahead in the rapidly evolving field of machine learning can be daunting, but it becomes manageable with the right resources. This article unveils a curated list of indispensable platforms that are regularly updated with the freshest insights from machine learning experts. Learn how to navigate the wealth of information and leverage expert knowledge to keep your skills sharp and informed.
- Social Media: AI Trends Gateway
- Papers with Code: Theory Meets Practice
- ArXiv.org: Cutting-Edge ML Research Hub
- Academic Papers: ML Engineering's Guiding Light
Social Media: AI Trends Gateway
In my view, social media remains the premier platform for discovering AI-related trends and developments within the industry. Actively participating in LinkedIn communities and engaging with groups on various social media platforms are excellent ways to stay informed about the latest AI advancements. Additionally, it's crucial to follow leading figures and top companies in the AI sector. These leaders often share insights and breakthroughs that can provide a deeper understanding of the field's direction. Following such influencers helps you grasp the practical applications of AI across different industries and the innovative solutions they offer. Given the dynamic nature of AI and its impact on technology, now is an opportune time to invest in understanding and leveraging AI developments.
Papers with Code: Theory Meets Practice
Honestly, my go-to is a combination: Papers with Code paired with the occasional deep-dive on Hugging Face's blog. That's the sweet spot between cutting-edge research and hands-on practicality.
Papers with Code is invaluable because it doesn't just present academic papers--it connects the dots by linking directly to GitHub repositories, metrics, and benchmarks. So instead of reading a dense paper and guessing how to implement it, you can see the actual code, test results, and where it fits in the bigger picture. It's like having the blueprint and the toolbox at the same time.
Then Hugging Face brings that extra layer of community and real-world use cases. Their blog breaks down bleeding-edge concepts in a way that doesn't feel like you need a PhD in mathematics to follow. You get this beautiful balance of theory and execution--which, let's be honest, is where the real learning happens.
When you're working in ML, you need signal over noise--and these two resources bring clarity without the fluff.
ArXiv.org: Cutting-Edge ML Research Hub
Staying on top of the latest advancements in machine learning engineering requires reliable and insightful resources, and one of my favorites is the website ArXiv.org. This platform hosts preprints of research papers from various fields including machine learning, making it a treasure trove of cutting-edge information before it's formally published. Researchers from around the world contribute their findings, giving readers insights into the very latest developments, often before they hit more mainstream journals or conferences.
What makes ArXiv particularly valuable is its accessibility and the breadth of topics it covers. You can explore papers on everything from fundamental algorithmic advances to applied uses of machine learning in fields like healthcare and autonomous driving. This allows practitioners not only to keep up with new algorithms and theory but also to see how these advancements can be applied in real-world scenarios. Plus, with its daily updates, there's always something new to learn. This constant stream of information encourages continuous learning and can be incredibly beneficial for anyone looking to refine their expertise or solve new challenges in the field of machine learning.
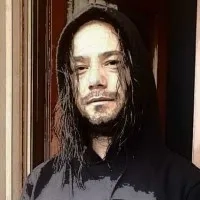
Academic Papers: ML Engineering's Guiding Light
To stay updated on machine learning engineering trends, academic research papers from reputable journals, conferences, and platforms like arXiv.org are invaluable. They provide insights into innovative algorithms and real-world applications directly from experts. Peer-reviewed studies help practitioners identify tested methodologies, enhancing their business strategies. For example, a case study on a predictive analytics model optimizing customer engagement in digital marketing illustrates practical applications.
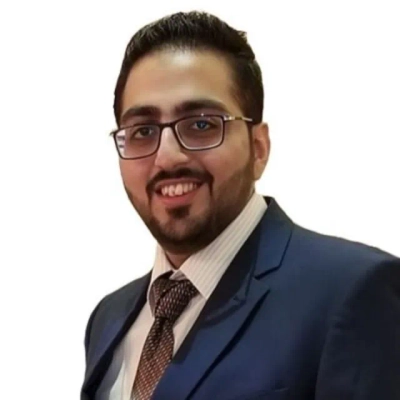