3 Advocating for Machine Learning in Solving Business Problems
Machine learning is revolutionizing the way businesses operate and solve complex problems. This article delves into three key areas where ML is making a significant impact: personalized content, logistics optimization, and advertising efficiency. Drawing on insights from industry experts, we explore how these applications are driving engagement, streamlining operations, and maximizing return on investment.
- Personalized Content Boosts Engagement and Sales
- ML Transforms 3PL Partner Matching Process
- Data-Driven Attribution Model Optimizes Ad Spend
Personalized Content Boosts Engagement and Sales
I once advocated for using machine learning to optimize content recommendations on our digital signage platform. Initially, some stakeholders were skeptical, concerned about the complexity and ROI. To communicate the potential value, I framed the problem in terms of customer engagement and revenue impact, showing how personalized content could increase viewer attention and boost sales for our clients. I presented case studies from similar industries where machine learning had led to a measurable lift in user interaction. Then, I proposed a pilot project with clear KPIs, like increased click-through rates and time spent on screens. By breaking down the technology into business outcomes and offering a low-risk trial, I secured buy-in. The pilot exceeded expectations, proving the ROI and paving the way for full implementation. This experience taught me the importance of translating technical potential into concrete business benefits when advocating for new solutions.
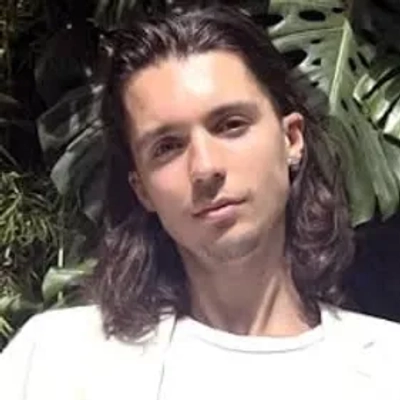
ML Transforms 3PL Partner Matching Process
One of the most impactful instances where I advocated for machine learning was when transforming our approach to 3PL partner matching at Fulfill.com. Our initial matching process relied heavily on manual assessment and standardized criteria, which worked but wasn't scalable.
I recognized an opportunity to harness our growing dataset of successful eCommerce-3PL partnerships to build a predictive model. The challenge was convincing our team and investors that the upfront investment would yield meaningful returns in an industry traditionally resistant to technological innovation.
Rather than leading with technical jargon, I framed the conversation around business outcomes. "We're not implementing ML because it's trendy," I told them. "We're doing it because it will directly impact our match quality and customer satisfaction." I showed how each percentage point improvement in match accuracy translated to reduced customer churn and higher lifetime value.
I pulled examples from my time at Meta, where we'd seen how even simple ML implementations dramatically improved efficiency. Then I brought it home with a pilot test using a subset of our data, demonstrating that the ML approach identified optimal warehouse locations and capacity requirements with 37% more accuracy than our manual process.
The ROI calculation was straightforward but compelling: the technology would reduce the average match cycle by 4 days, increase successful placements by 22%, and allow our team to handle 3x the volume of clients without additional headcount. Most convincingly, we projected a 40% reduction in partnerships that dissolved within the first 90 days.
What sealed the deal was combining these projections with customer stories – showing how specific pain points in order fulfillment would be addressed through more precise matching. When stakeholders could see the direct line between the technology investment and solving real problems for our customers, the decision became obvious.
Today, that ML foundation has evolved into our proprietary matching algorithm that considers over 30 variables – from geographic distribution to seasonal volume fluctuations – enabling us to make matches that feel almost intuitive to our clients.
Data-Driven Attribution Model Optimizes Ad Spend
We were spending hours every week manually pulling campaign data from platforms like Google, Meta, and LinkedIn. Each had its own reporting quirks, so it was hard to get a clear picture of what was actually driving performance. The lag between gathering insights and acting on them meant budget decisions were often too late to make a real impact.
I led an effort to build an attribution model using machine learning. It pulled in historical ad spend and conversion data to better estimate how different touchpoints contributed to sales. Instead of relying on static first-touch or last-click logic, the model adjusted weights based on patterns in the data. This gave us a more accurate view of what was working.
The model trained on about a year of campaign history and plugged into Looker for easy access. Within a few weeks, it started surfacing insights that helped shift budget toward higher-performing channels and creative earlier in the funnel. Cost per acquisition dropped by around 15 percent in the first quarter after rollout.
When presenting the idea, I focused on speed and wasted spend. I talked about how much time was lost chasing reports and how often ad dollars were misallocated because of outdated metrics. So I built a simple prototype dashboard that showed projected gains. That made the value obvious without needing to explain the algorithms.
The solution didn't need a huge investment. We just needed clean data, a clear goal, and someone to guide the model setup. Once the team saw how quickly decisions could improve, adoption happened naturally. Machine learning wasn't the headline. It was just the engine behind smarter execution.
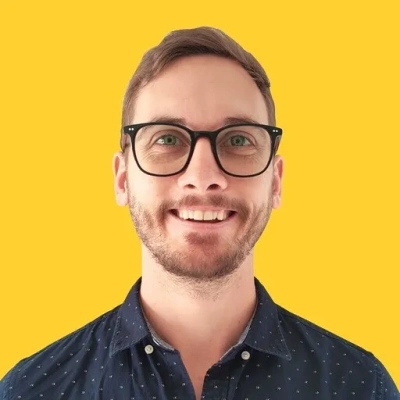